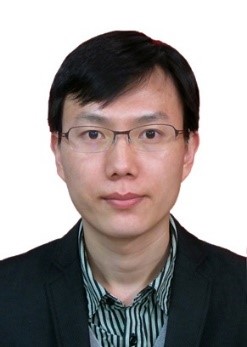
Short Bio:
Zai Yang is a Professor of the School of Mathematics and Statistics, Xi’an Jiaotong University, China. He received the B.Sc. degree in mathematics and M.Sc. degree in applied mathematics from Sun Yat-sen (Zhongshan) University, China, in 2007 and 2009 respectively, and the Ph.D degree in electrical and electronic engineering from Nanyang Technological University (NTU), Singapore, in 2014. He is an IEEE Senior Member and serving on the editorial board of Signal Processing (Elsevier). His research interests include compressed sensing and optimization theory and their applications in signal and information processing, big data analytics, and machine learning. He was awarded the NSFC Excellent Youth Science Foundation Grant in 2019.
Title: Low-rank optimization methods for spectral compressed sensing
Abstract: Spectral compressed sensing refers to the recovery of a spectrally sparse signal from compressive measurements and has wide applications in radar and wireless communications. It is linked to low-rank matrix recovery by applying Kronecker and Carathéodory-Fejér theorems for Hankel and Toeplitz matrices, based on which convex and nonconvex optimization algorithms have been proposed. In this talk, we will introduce previous low-rank matrix recovery formulations and point out their limitations. After that, we present two new low-rank optimization models to resolve these limitations and demonstrate their effectiveness with convex and nonconvex algorithms.
|