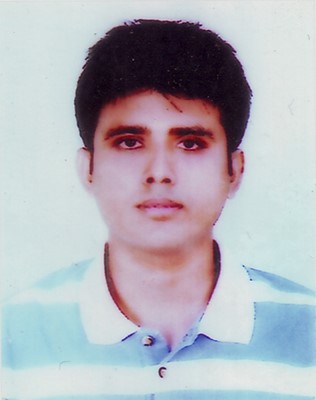
ABDULLAH-AL NAHID received the B.Sc. degree in Electronics and Communication Engineering from Khulna University, Khulna, Bangladesh, in 2007, the M.Sc. degree in telecommunication engineering from the Institute for the Telecommunication Research (ITR), University of South Australia (UniSA), Australia, in 2014, and the Ph.D. degree from Macquarie University, Sydney Australia, in 2018. His research interests include machine learning, biomedical image processing, data classification, and biomedical data analysis. He is a passionate researchers. He has published more than eighty journal articles, conference proceeding. Along with this he has published four book chapters. Due to his outstanding research activities, he has awarded Vice-Chancellor Award's by Khulna University, Khulna-9208, Bangladesh in 2021.
Title: Machine Learning and its uses in Human Action Recognition
Abstract: Human activity recognition (HAR) is the process of automatically determining a person's activity based on sensor data. HAR is used in a wide range of industries, such as healthcare, sports, security, and human-computer interaction. The use of HAR allows for the tracking of elderly or disabled individuals' movements and the detection of any unusual behavior. It can be used in offices to monitor worker movement and identify potential risks like injuries from repetitive motion. Security applications that use HAR can alert authorities right away to any suspicious activity. HAR systems typically use sensors like accelerometers, gyroscopes, and magnetometers in devices such as smartphones, smart-watches, and fitness trackers to collect data on human movement. Machine Learning (ML) algorithms can then examine the data to identify actions like standing, sitting, and running. For HAR tasks, ML techniques are frequently employed. CNNs are frequently used in tasks involving image and video analysis, and they have also been used in HAR. RNNs can detect temporal dependencies in sensor data because they are made to handle sequential data. RNNs have been used in HAR tasks like gesture recognition where the order of sensor data is crucial. Long Short-Term Memory’s (LSTM) have been used for HAR tasks like identifying a series of physical exercises where the length of an activity varies. In HAR tasks, where the learned features are fed into a classification model, autoencoders can be used for feature extraction. Overall, HAR has a wide variety of uses in the human world, from security and home automation to sports and healthcare. We can anticipate seeing even more cutting-edge uses as deep learning-based HAR techniques develop. |